Adding to the omnipresent discussion of Artificial Intelligence (AI), this paper examines whether spiking US venture capital developed into a dotcom-like AI bubble. Drawing from economic theory on bubbles, the paper first outlines the “investment bubble mechanism” and the “bubble spread”, before demonstrating boom and bust at the example of the 1999/2000 dot-com bubble. Analysing venture capital, patent, and stock market data from 1990 to 2018, the paper finds little evidence for an AI-related bubble. The AI hype does not drive overall venture capital development, let alone stock market indices like NASDAQ Composite or the Dow Jones Industrial Average. Even the AI-specific venture capital, which skyrocketed in the past 5 years and lead to bubble concerns, seems substantially backed by profitability projections and patents numbers. To avoid throwing all caution of AI bubbles to the winds, the paper recommends two policy approaches to take advantage of the development and avoid harmful future bubble spread.
“Neural Network”, “Intelligent Systems”, “Predictive Analytics”, “Machine Learning”, “Natural Language Processing”, and “Deep Learning” – all these names sum up under Artificial intelligence (AI) and have befallen public discussions, policy analysis, and investment calculations. Based on increasing speed of technological development in AI, research and investment intensified exponentially. Especially venture capital in AI skyrocketed (Rowley, 2018). On the other side of the hype, analysts are calling for reluctance. They point out the still limited abilities of AI and the negative effects of overhyping its soon-might-have potential (Greene, 2018; Middleton, 2018).
This paper takes up the warning and examines the current AI investment from the economic bubble perspective. Comparing the AI hype to the much-discussed dot-com bubble at the turn of the millennium, this paper answers two related questions: First, has venture capital employment in AI developed into unbacked, hyped investment, where expected returns are considerably at variance from intrinsic returns (bubble mechanism)? Second, has the investment bubble spread to a potentially harmful asset bubble, driving overall venture capital and stock market development (bubble spread)? In the following, the paper briefly introduces theoretical arguments on the economic bubbles, demonstrates how the spread level of the dot-com bubble harmed stock markets and economic development, and finally answers the above-mentioned questions regarding a potential AI bubble. Two policy recommendations, based on the paper’s evaluation, serve as final remarks.
Theory of investment bubbles: Bubble Mechanism and Bubble Spread
Bubble Mechanism
Throughout history, economies all over the world experienced bubbles: Tulip mania in the Netherlands (17th century), British South Sea bubble in the 18th century, Japanese asset price bubble (1986-1991), the dot-com bubble (USA; 1995-2000), 2000s property bubbles in Spain, Ireland or Australia, and most recently, the Cryptocurrency bubble (2011-2018). All bubbles share a “bubble mechanism”. For the most common type of bubble, the asset bubble, this mechanism consists of “trade in high volumes at prices that are considerably at variance from intrinsic values” (Garber, 1990; King, Smith, Williams, & Van Boening, 1993; Levine & Zajac, 2007). The intrinsic value is a theoretical approach to calculate fair, future profitability based on potential benefits and investment risks, disregarding gains through speculative behaviour in multiple-actor scenarios (Girdzijauskas et al., 2009; King et al., 1993). The more prices deviate from the intrinsic value, the greater the bubble. Comparing different types of bubbles (Girdzijauskas et al., 2009), this paper highlights the difference between asset and investment bubbles. Asset price bubbles, such as the Japanese asset price or 2000s property bubble, are defined by their above mentioned bubble mechanism and rely on the speculative rationale of being able to resell asset for a higher price later (Barlevy, 2011). Investment bubbles, on the contrary, require an estimate of future returns and implied risks on their investments so that the funded project pays off (Girdzijauskas et al., 2009). Their bubble mechanism adapted to investment bubbles could be phrased as: The investment bubble mechanism is the investment in high volumes at expected returns on investment that are considerably at variance from the intrinsic returns on investment.
The differentiation in rationales matters: As asset bubbles are relatively dependent on the call (buy) or put (sell) price, investment bubbles are substantively linked to the features of the underlying investment (Rigotti, Ryan, & Vaithianathan, 2016). Therefore, investment bubbles are especially vulnerable to uncertainty regarding their future pay-off (Evanoff, Kaufman, & Malliaris, 2012). The malicious element of an asset bubble lies in finite (instead of infinite) demand for hyped assets, whereas the pitfall of investment bubbles lies in the uncertainty about future success rates and the hence wrong assessment of its profitability (DeMarzo, Kaniel, & Kremer, 2007).
Bubble Spread
To examine the potential threat of an AI investment bubble for technological innovation as well as macroeconomic stability, this paper evaluates the “bubble spread”, i.e., supportive circumstances for asset bubble formation, and the stage of development from investment to asset bubble. The theory section closes with brief remarks on the potential harms of spread investment bubbles.
Puzzled by the question of what exactly causes economic bubbles, scholars stressed supportive circumstances for (asset) bubble formation, such as high liquidity, low interest rates, and socio-psychological factors. The excessive provision liquidity and the lack of deployment possibilities encourage more risk-taking behaviour as capital is available but low-risk investment opportunities are lacking (Acharya & Naqvi, 2012; Porras, 2016). Low interest rates that create excessive liquidity further encourage the shift in risk structure as non-risky investment options are not only scarce but pay off less (Christiano, Ilut, Motto, & Rostagno, 2010). Paul Krugman (2015) summarized excess liquidity and bubbles splendidly: “What we’re seeing is what happens when too much money is chasing too few investment opportunities.” Beyond monetary policy, also socio-psychological factors contribute to the emergence of asset bubbles (and hence facilitate the spread from investment to asset bubble). Teeter & Sandberg (2017) outline the importance of a stock market narrative that incorporates “extrapolation” (assuming future development to be a continuation of previous experiences) and “herding” (orientation of individual behaviour along the market trend). Both bubble effects appear as a reflex to uncertainty, providing a sense of safety in the community (herding) (DeMarzo et al., 2007) and maintain the status quo bias (extrapolation) (Barberis, Greenwood, Jin, & Shleifer, 2018).
In the context of high liquidity, low interest rates, and “bubbly” socio-psychological factors, it is key to understand the relationship between investment and asset bubble: an asset bubble may develop out of the investment bubble, but not every asset bubble relies on an investment bubble. For example, the dot-com bubble spread to an asset bubble (see next section; Schularick, Taylor, & Jordà, 2015); yet, the South Sea or Japanese asset bubble have not been anteceded by investment bubbles (Garber, 1990). The transfer from an investment into an asset bubble is encouraged by companies maturing and shifting their investment scheme from venture capital to stock market funding, e.g. through an IPO exit. The investment logic behind the now tradable assets changes: Not the risk-benefit calculation of the business matters anymore but whether the later selling price exceeds purchase price. Supportive monetary and socio-psychological factors accelerate speculative behaviour of stock market traders if capital is unemployed, credit cheap and low-risk investment opportunities limited. Two distinct indicators signal the malicious spread from investment to asset bubble: First, the large-scale entrance of private investors and “bubble riding” of hedge funds (Griffin, Harris, Shu, & Topaloglu, 2011). Second, bubble assets driving the development of aggregate stock market indices, such as NASDAQ Composite, TecDAX, or Dow Jones Industrial Average.
As the stock market is more sensitive to profitability volatility than venture capital, defaulting start-ups may threaten sector stability. This is especially the case when the sector is small and speculation high. If profitability of these young companies fluctuates, a spread investment bubble (which than work like an asset bubble) can trigger a stock market landslide, leading to the bubble bust. This anon may trigger economic decline via defaulted credits, a cutback in credit supply, reduced demand for assets, falling prices, etc. (Barlevy, 2018). Depending on its characteristics (esp. leverage and credit structure), the spread investment bubble can result in economic recession/depression as seen after the dot-com, the 1929 or the 2008 bubbles (Schularick et al., 2015). In addition, there is an investment-specific risk, even before the bubble spread: If hyped expectation on venture capital are disappointed, the herded stop of investments might harm the innovation in the whole sector and potentially lead to a so-called innovative winter. A well-known example is the stopping investment in British railways after the bust of the Railway mania bubble in the 1850s (Bryer, 1991).
The Dot-Com Investment Bubbles: Bubble Spread in Practice
The case of the dot-com bubble, a prototype investment bubble in the sense of DeMarzo, Kaniel, & Kremer (2007), serves as an example to illustrate the development from a hyped investment into a malicious asset bubble. The paper outlines the bubble mechanism and the bubble spread, before briefly describing the burst in April 2000
Starting with a rush to the internet, investments in computer- and internet-based innovation accelerated from mid 1990s on (Kline, 2003; U.S. Department of Labor, 1999). While US venture capital rose steadily from 1995 to end 1998, the investment almost quadrupled from US$ 7.2 bn in the first quarter of 1991 to US$ 32.2 bn a year later (see figure 1). This unprecedented boom spread to the stock market as tech-related IPOs rose over the 1990s and peaked in 1999/2000 not only in absolute terms (1999: 370; 2000: 260 tech IPOs) but also in its relative share to non-tech IPOs (74 % of all IPOs were tech-related, in comparison to 33 % from 1990 to 1999, see figure 2). It is therefore no wonder that tech-oriented NASDAQ Composite mimicked the VC development (see figure 3). Within a year, NASDAQ Composite doubled its index points and the Dow Jones Industrial Average profited from the economic boom, too. As the hype turned into a mania and private investors as well as hedge funds started to enter to enter the market in large numbers (Griffin et al., 2011), IPO over-pricing contributed majorly to the sharp increase in stock market indices (Kayne & Laux, 2005; Ljungqvist & Wilhelm, 2003).
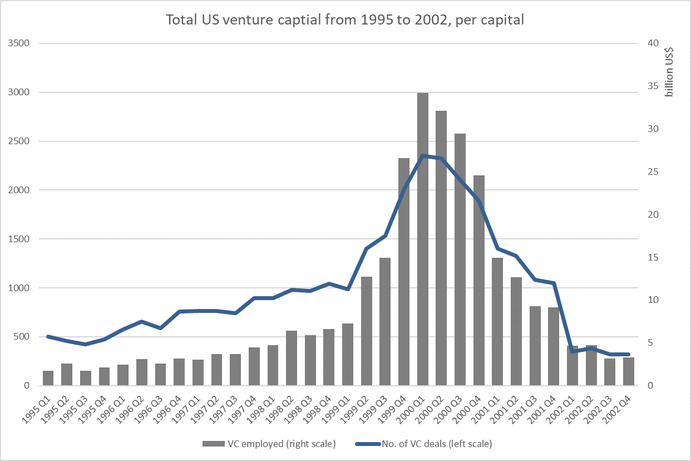
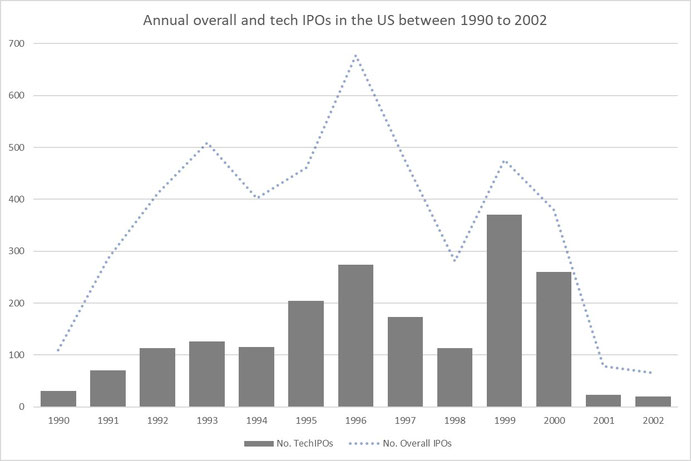
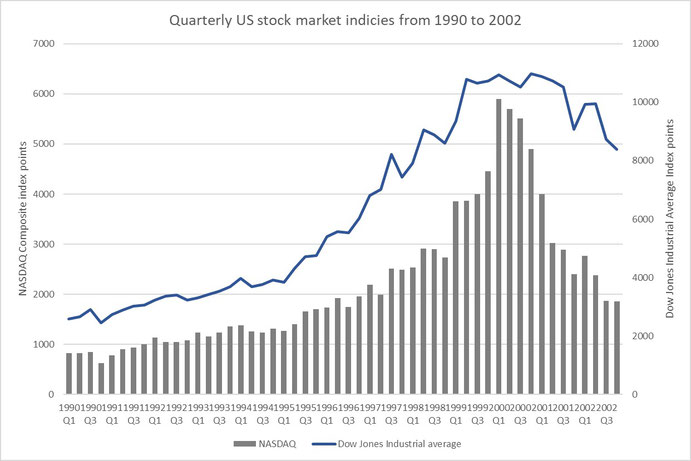
With a shift from investment to asset bubble logic, investors required rising share prices to keep their trading profitable. This increased pressure on the profitability of public dot-com companies and stress in the markets. From March 2000 on, a series of events that indicated the lack of profitability of over-capitalized start-ups shook the market: “Yahoo!” and “eBay” merger talks failed, a few days later “MicroStrategy” announced a revenue restatement due to aggressive accounting, and in April “Microsoft” was ruled guilty of monopolization and tying in an anti-trust law case on federal level (Alden, 2005). Facing decreasing stock market value, the bankruptcies of start-ups only few months after their IPO finally triggered the burst of the bubble (Basco, 2018; Morris & Alam, 2008). Until mid-2002, NASDAQ Composite shrank by 70 %, tech and non-tech IPOs almost disappeared, and the venture capital market collapsed to pre-1996 level. As the downturn spread to the Dow Jones and resulted in negative GDP growth, the dot-com bubble drove the United States into a recession (Kleinbard, 2000; NBER, 2009).
Regarding the supportive environment for the spread from investment to asset bubble from an ex-post perspective, monetary circumstances with moderate, even increasing, interest rates and rising but not excessive credit growth have not boosted the bubble additionally (Federal Reserve data, see Appendix A). Yet, the socio-psychological factors stood out: Herding in the dot-com bubble serves as prime example of a trend turning into a hype, and a hype into a mania, as many scholars assessed (DeMarzo et al., 2007; Lindhe, 2012; Marcos-Prieto & Perote, 2017; Singh, 2013). In addition, extrapolation (the assumption of future development to be a continuation of previous experiences) also contributed to the bubble (Barberis et al., 2018).
Assessing Bubble Mechanism and Spread: Is the US running into an AI bubble?
Observing the development of innovation and investment in Artificial Intelligence, references to the dot-com bubble seem inevitable: Not only is AI technology based on the internet and frequently referred to companies like Google or Amazon that emerged from the dot-com bubble, but public discussion, investors obsession, and employees’ apprehension hype the topic. Referring to seemingly comparable VC growth rates in the late 1990s, analysts point out the skyrocketing venture capital in AI start-ups nowadays (see, for example, Greene (2018), Middleton (2018), Rowley (2018), Walch (2018)). They concordantly ask: “Is the US running into a dotcom-like AI bubble?” This question consists of two sub-question: First, is the current hype seen in AI VC provoking an “AI winter” – the sudden stop of innovation in the sector? Second, has AI investment created an asset bubble that is likely to burst if expectations are not met? To proceed, the paper first addresses the Bubble Mechanism before turning to the Bubble Spread.
Bubble Mechanism: Is there an AI investment bubble?
While economists, analysts and even politicians agree on the potentials of Artificial Intelligence (thus fuelling expectations), the impact on businesses is still ambiguous (Brynjolfsson & Mitchell, 2017; Bughin et al., 2017; Perez, Deligianni, Ravi, & Yang, 2018). Venture capital investors specialise on this type of innovative uncertainty, adapting their ambiguity-risk-benefit profile to the expected profitability of a start-up (Grieco, 2018; Rigotti et al., 2016; Zhang, 2015). Regarding the sharp increase in venture capital invested in AI start-ups over the past four years (see figure 4), the aggregate success of the VC profitability calculation becomes a watershed for the sector (and – depending on the Bubble Spread – also for the overall economy). The more capital invested, the higher the expectation on future turnout; hence, the greater the risk of a sudden stop of investment when expectations are not met.
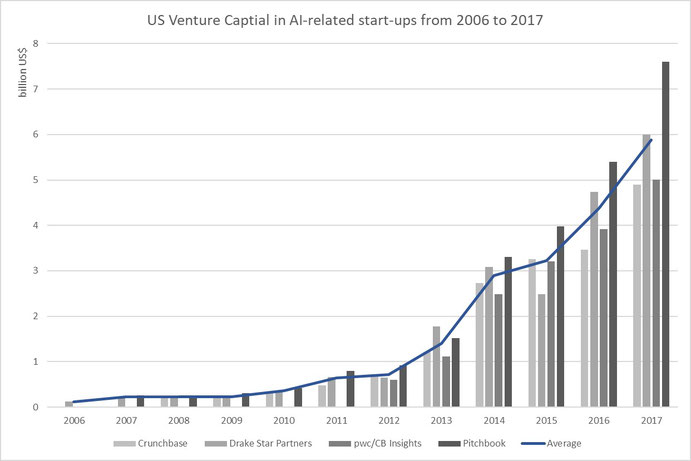
Yet, there is twofold evidence for an economic backing of AI profitability: First, apart from frequent anecdotal reports of the successful acquisition of AI start-ups, such as “Deepmind” by Google (US$ 400 mn) or “Turi” by Apple (US$ 200 mn) (Deagon, 2016), “Drake Star Partners”, a global investment banking firm, projected annual revenue growth rates of US AI companies to increase by 39.5 % until 2025 (Bedrosian & Finkler, 2018). Second, as VC grows, so does AI research and admission of patents (Fujii & Managi, 2018; Saravia, Slen, & Pendse, 2018). Following the clear link between a company’s intellectual capital and its profitability (see, for example, De Rassenfosse (2012), Ernst, Conley, & Omland (2016), or Sydler, Haefliger, & Pruksa (2014)), US patents mimcry the rise of AI VC and serve as an additional argument to the profitability of the AI sector (see figure 5).
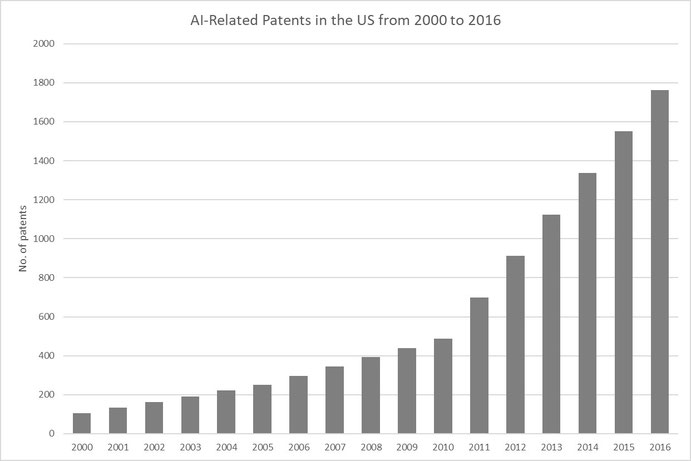
Furthermore, not only the venture captial investment rose over the past four years, but so did government spending and corporate R&D investment in the field. In the US, government spending increased by 40 % since 2015 to approx. US$ 1 bn in 2017 (The White House, 2018); a even larger share came from already existing big players in the field: Google, Amazon, IBM, Mircosoft, Facebook, Apple, among others, spend approx. US$ 15 bn on AI R&D in the US (European Commission, 2018). Finally, scholar, who may discuss consequences of Aritificial Intelligence controversely, unanimously agree that AI, and in particular Machine Learning, is the general purpose technology of the 21st century, further manifesting the strong stance of the sector (Brynjolfsson, Rock, & Syverson, 2017).
Against the vivid recollection of venture captial at the center of the dot-com bubble, the substantial backing of AI investment through patents and profitability projections, as well as big companies’ and even governments’ interest in the technology, make a sudden stop of investments and especially an AI winter highly unlike.
Bubble Spread: Is there an AI investment-based asset bubble?
Regarding the second sub-question, the previous argumentation has not yet addressed the level of spread from investment to asset bubble. In this case, the speculative logic of stock market investors would increasese already high expectations on AI; additionally, not the aggregate profitability of the sector in the balanced VC calculation but single, expectation-hampering company defaults can trigger a stock market landslide and a bubble burst.
The AI hype falls in a time of low interest rates and high credit growth (see Appendix A), as well as supportive socio-psychological factors, such as herding and extrapolation, which are both fuelled by the high uncertainty in the sector (Köhn, 2018). The circumstances provide an ideal basis for the spread from investment to asset bubble; yet, little can be observed. The sharp increase in AI venture capital between 2012 and today is only partially detectable in the overall venture capital development in the US (see figure 6). Compared to the dot-com spike of approx. 74 % VC increase per quarter, recent increase of approx. 14 % per quarter appears moderate. Even though NASDAQ composite showed considerable growth since the Great Recession and there are warning about the next “normal” asset bubble (see figure 8; Colombo, 2018), this increase cannot be linked to the spread from an VC investment to an asset bubble. The reason for this lies in missing IPOs of AI start-ups: In contrary to the dot-com bubble, almost all AI start-ups exit via acquisition, not by IPO, selling their innovation to one of the previously described big players in the sector instead of entering the stock market (The Economist, 2017). In 2018, no US start-up exited by IPO so far, whereas 43 start-ups were acquired (Venture Scanner, 2018).
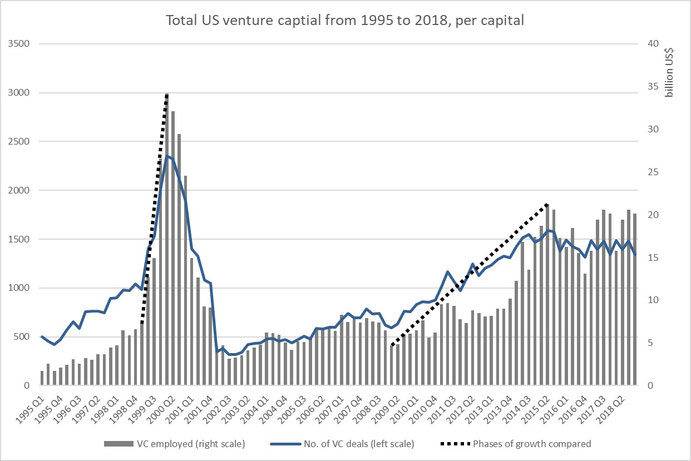
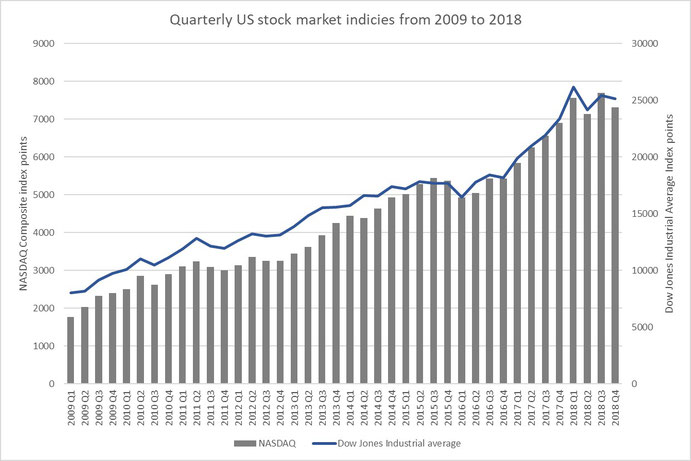
Though raising concerns of monopolization, big companies, such as Google, IBM, or Qualcomm, reduce stock market volatility as they can balance unprofitable acquisition with other streams of revenue through the so-called “size premium” (Asness, Frazzini, Israel, Moskowitz, & Pedersen, 2018; Hou & Van Dijk, 2018). As the multinationals’’ stock market price only very partially relies on the acquired innovation, the acquisition instead of an IPO reduces harmful stock market volatility and cushions AI expectations.
Conclusion
With almost no IPOs of AI start-ups and no distinct trend in overall VC or stock market indices, there is little evidence for a spread from a VC investment to an asset bubble. Furthermore, the sharp increase in AI-related venture capital is economically backed by projected revenue growth and more patents. The AI hype has therefore not (yet) resulted in a dotcom-like investment or asset bubble. Nevertheless, the spiking development in AI is prone to some relaxation, anticipated by some VC analysts (Rowley, 2018). As soon as venture-backed start-ups turn out unprofitable, expectations will most likely flatten, and a market cleansing effect appears. Due to the strong stance of big companies and governments’ interest in the field, it seems implausible that this downturn results in a sudden stop or AI winter.
To avoid throwing all caution to the winds, the paper recommends two approaches: First, policy makers and economists should appreciate the current positive effects of increasing investment in AI. Hyped and partially overly liquid investments allow start-ups to venture innovative paths that previous funding constraints inhibited (Sorescu, Sorescu, Armstrong, & Devoldere, 2018). As Schnitzer and Watzinger (2017) outline, these innovation are not limited to the start-ups but likely spill over to other companies in the sector. In the current situation with almost not stock market capitalization of AI start-ups, the AI hype supports innovation in the sector. Even if the profitability calculation is further stressed and VC investment becomes “bubblier”, authors like Martin and Ventura (2011, 2018) argue that the investments in innovation still outweigh bubble risks as they further overall growth. The paper therefore proposes to support the innovation in AI-related, venture-backed start-ups as long as Bubble Spread stays limited.
Second, in the current monetary setting of low (yet, increasing) interest rates and high credit growth, policy makers should observe IPO exits and the stock market capitalization of AI start-ups. This is particularly of interest, if policy makers assess anti-trust measures against AI-investing multinationals, like Google, Amazon, or Qualcomm. Here, the de-monopolization can discourage start-up acquisition and foster IPOs. This possible shift towards the stock market accelerates the spread from investment to asset bubble.
This research was submitted as final paper for the course "Advanced Economics: Economic Theory & Policy" at Hertie School of Governance, lectured by Prof Jean Pisani-Ferry, Chief Economist of the French President and Professor at Hertie School of Governance and SciencePo.
Appendix: Fed Data
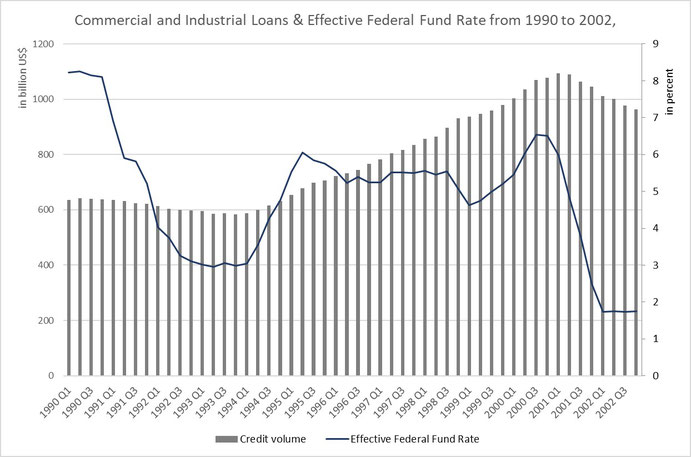
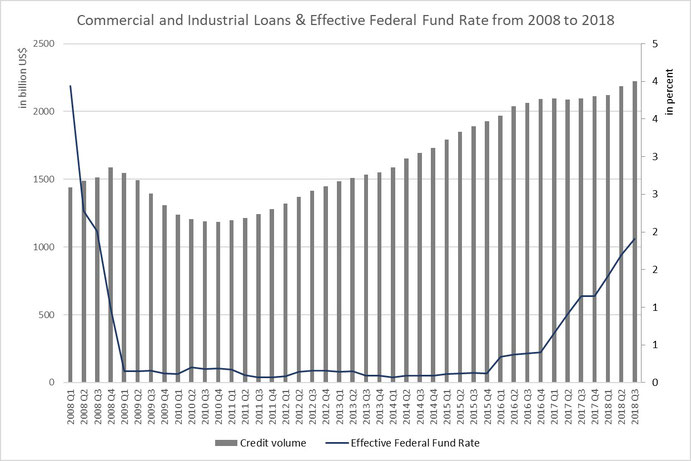
LITERATURE
- Acharya, V., & Naqvi, H. (2012). The seeds of a crisis: A theory of bank liquidity and risk taking over the business cycle. Journal of Financial Economics, 106(2), 349–366. https://doi.org/10.1016/j.jfineco.2012.05.014
- Alden, C. (2005). Looking back on the crash | Technology | The Guardian. Retrieved December 12, 2018, from https://www.theguardian.com/technology/2005/mar/10/newmedia.media
- Aliber, R. Z., & Kindleberger, C. P. (2017). Manias, panics, and crashes: A history of financial crises, seventh edition. Manias, Panics, and Crashes: A History of Financial Crises, Seventh Edition. https://doi.org/10.1007/978-1-137-52574-1
- Asness, C., Frazzini, A., Israel, R., Moskowitz, T. J., & Pedersen, L. H. (2018). Size matters, if you control your junk. Journal of Financial Economics, 129(3), 479–509. https://doi.org/10.1016/j.jfineco.2018.05.006
- Barberis, N., Greenwood, R., Jin, L., & Shleifer, A. (2018). Extrapolation and bubbles. Journal of Financial Economics, 129(2), 203–227. https://doi.org/10.1016/j.jfineco.2018.04.007
- Barlevy, G. (2011). Economic theory and asset bubbles. Economic Perspectives, Q3(Q III), 44–59. Retrieved from http://ideas.repec.org/a/fip/fedhep/y2007iqiiip44-59nv.31no.3.html
- Barlevy, G. (2018). Bridging between policymakers’ and economists’ views on bubbles. Economic Perspectives, (4). https://doi.org/https://doi.org/10.21033/ep-2018-4
- Basco, S. (2018). A Brief History of Bubbles. In Housing Bubbles: Origins and Consequences (pp. 5–15). Cham: Springer International Publishing. https://doi.org/10.1007/978-3-030-00587-0_2
- Bedrosian, G., & Finkler, L. (2018). Industry Update: Artificial Intelligence - North America.
- Bryer, R. A. (1991). Accounting for the “railway mania” of 1845- A great railway swindle? Accounting, Organizations and Society. https://doi.org/10.1016/0361-3682(91)90038-G
- Brynjolfsson, E., & Mitchell, T. (2017). What can machine learning do? Workforce implications. Science Magazine, 358(6370), 1530–1534. https://doi.org/10.1109/ARSO.2005.1511626
- Brynjolfsson, E., Rock, D., & Syverson, C. (2017). Artificial Intelligence and the Modern Productivity Paradox: A Clash of Expectations and Statistics (NBER Working Paper series No. 24001). https://doi.org/10.3386/w24001
- Bughin, J., Hazan, E., Ramaswamy, S., Chui, M., Allas, T., Dahlström, P., … Trench, M. (2017). Artificial Intelligence: the Next Digital Frontier?
- Christiano, L., Ilut, C. L., Motto, R., & Rostagno, M. (2010). Nber Working Paper Series Monetary Policy and Stock Market Booms. Retrieved from http://www.nber.org/papers/w16402
- Colombo, J. (2018). Disaster Is Inevitable When America’s Stock Market Bubble Bursts. Retrieved December 13, 2018, from https://www.forbes.com/sites/jessecolombo/2018/09/05/disaster-is-inevitable-when-americas-stock-market-bubble-bursts/#2e4922e71b82
- de Rassenfosse, G. (2012). How SMEs exploit their intellectual property assets: Evidence from survey data. Small Business Economics, 39(2), 437–452. https://doi.org/10.1007/s11187-010-9313-4
- Deagon, B. (2016). AI Meets ROI: Where Artificial Intelligence Is Already Smart Business | Investor’s Business Daily. Retrieved December 12, 2018, from https://www.investors.com/news/technology/artificial-intelligence-weaves-its-way-deeper-into-daily-business/
- DeMarzo, P., Kaniel, R., & Kremer, I. (2007). Technological innovation and real investment booms and busts. Journal of Financial Economics, 85(3), 735–754. https://doi.org/10.1016/j.jfineco.2006.07.003
- Ernst, H., Conley, J., & Omland, N. (2016). How to create commercial value from patents: the role of patent management. R and D Management. https://doi.org/10.1111/radm.12210
- European Commission. (2018). USA-China-EU plans for AI: where do we stand? Retrieved from https://ec.europa.eu/growth/tools-databases/dem/monitor/sites/default/files/DTM_AI USA-China-EU plans for AI v5.pdf
- Evanoff, D. D., Kaufman, G. G., & Malliaris, A. G. (2012). Chicago Fed Letter (Chicago Fed Letter: Essays on Issues No. 304). Chicago.
- Fujii, H., & Managi, S. (2018). Trends and priority shifts in artificial intelligence technology invention: A global patent analysis. Economic Analysis and Policy, 58, 60–69. https://doi.org/10.1016/j.eap.2017.12.006
- Garber, P. M. (1990). Famous First Bubbles. Journal of Economic Perspectives. https://doi.org/10.1257/jep.4.2.35
- Girdzijauskas, S., Štreimikiene, D., Čepinskis, J., Moskaliova, V., Jurkonyte, E., & Mackevičius, R. (2009). Formation of economic bubbles: Causes and possible preventions. Technological and Economic Development of Economy, 15(2), 267–280. https://doi.org/10.3846/1392-8619.2009.15.267-280
- Greene, T. (2018). What happens when the AI bubble bursts? Retrieved November 12, 2018, from https://thenextweb.com/artificial-intelligence/2018/06/13/what-happens-when-the-ai-bubble-bursts/
- Grieco, D. (2018). Innovation and stock market performance: A model with ambiguity-averse agents. Journal of Evolutionary Economics, 28(2), 287–303. https://doi.org/10.1007/s00191-017-0537-1
- Griffin, J. M., Harris, J. H., Shu, T., & Topaloglu, S. (2011). Who drove and burst the tech bubble? Journal of Finance, 66(4), 1251–1290. https://doi.org/10.1111/j.1540-6261.2011.01663.x
- Hou, K., & Van Dijk, M. A. (2018). Resurrecting the Size Effect: Firm Size, Profitability Shocks, and Expected Stock Returns. Ssrn. https://doi.org/10.2139/ssrn.1536804
- Kayne, D., & Laux, J. (2005). IPO Returns : Pre And Post Dotcom Bubble, 3(11), 1–6. https://doi.org/10.19030/jber.v3i11.2822
- King, R. R., Smith, V. L., Williams, A. W., & Van Boening, M. V. (1993). The robustness of bubbles and crashes in experimental stock markets. Nonlinear Dynamics and Evolutionary Economics, (August), 183–200. https://doi.org/10.1016/j.neuroscience.2013.11.014
- Kleinbard, D. (2000). Dot.coms lose $1.755 trillion in market value - Nov. 9, 2000. Retrieved December 12, 2018, from https://money.cnn.com/2000/11/09/technology/overview/
- Kline, G. (2003). Mosaic started Web rush & Internet boom | News-Gazette.com. Retrieved December 11, 2018, from http://www.news-gazette.com/news/local/2003-04-20/mosaic-started-web-rush-internet-boom.html
- Köhn, A. (2018). The determinants of startup valuation in the venture capital context: a systematic review and avenues for future research. Management Review Quarterly, 68(1), 3–36. https://doi.org/10.1007/s11301-017-0131-5
- Krugman, P. (2015). A Moveable Glut - The New York Times. Retrieved December 10, 2018, from https://www.nytimes.com/2015/08/24/opinion/a-moveable-glut.html?action=click&pgtype=Homepage&module=opinion-c-col-left-region®ion=opinion-c-col-left-region&WT.nav=opinion-c-col-left-region
- Levine, S. S., & Zajac, E. J. (2007). The Institutional Nature of Price Bubbles. SSRN. https://doi.org/10.2139/ssrn.960178
- Lindhe, E. (2012). Herd Behavior in Stock Markets, (August), 1–52.
- Ljungqvist, A., & Wilhelm, W. J. (2003). IPO Pricing in the Dot-com Bubble. Journal of Finance, 58(2), 723–752. https://doi.org/10.1111/1540-6261.00543
- Marcos-Prieto, P., & Perote, J. (2017). Agents’ Behavior in Market Bubbles: Herding and Information Effects. Economics World, 5(1), 44–51. https://doi.org/10.17265/2328-7144/2017.01.005
- Martin, A., & Ventura, J. (2011). Economic Growth with Bubbles. NBER Working Paper. Retrieved from http://papers.ssrn.com/sol3/papers.cfm?abstract_id=1583811
- Martin, A., & Ventura, J. (2018). The Macroeconomics of Rational Bubbles: A User’s Guide. Annual Review of Economics, 10(1), 505–539. https://doi.org/10.1146/annurev-economics-080217-053534
- Middleton, C. (2018). AI bubble set to burst, says critical analyst report | Internet of Business. Retrieved November 12, 2018, from https://internetofbusiness.com/ai-bubble-set-to-burst-says-critical-analyst-report/
- Morris, J. J., & Alam, P. (2008). Analysis of the Dot-Com Bubble of the 1990s. Ssrn, (June). https://doi.org/10.2139/ssrn.1152412
- NBER (2009). US Business Cycle Expansions and Contractions. Retrieved December 12, 2018, from https://www.nber.org/cycles/cyclesmain.html
- Perez, J. A., Deligianni, F., Ravi, D., & Yang, G.-Z. (2018). Artificial Intelligence and Robotics. UK Robotics & Autonomous Systems Network.
- Porras, E. (2016). Bubbles and Contagion in Financial Markets, Volume 1: An Integrative View. Springer.
- Rigotti, L., Ryan, M., & Vaithianathan, R. (2016). Throwing good money after bad. Decisions in Economics and Finance, 39(2), 175–202. https://doi.org/10.1007/s10203-016-0183-3
- Rowley, J. D. (2018). Venture Funding Into AI And Machine Learning Levels Off As Tech Matures. Retrieved November 12, 2018, from https://news.crunchbase.com/news/venture-funding-ai-machine-learning-levels-off-tech-matures/
- Saravia, S., Slen, E., & Pendse, G. (2018). Artificial Intelligence & Robotics: Industry Report & Investment Case.
- Schnitzer, M., & Watzinger, M. (2017). Measuring Spillovers of Venture Capital (CEPR Discussion Paper No. 12236). Discussion Papers.
- Schularick, M., Taylor, A. M., & Jordà, Ò. (2015). Leveraged Bubbles. Journal of Monetary Economics.
- Singh, V. (2013). Did institutions herd during the internet bubble? Review of Quantitative Finance and Accounting, 41(3), 513–534. https://doi.org/10.1007/s11156-012-0320-1
- Sorescu, A., Sorescu, S. M., Armstrong, W. J., & Devoldere, B. (2018). Two Centuries of Innovations and Stock Market Bubbles. Marketing Science, 37(4), 507–684. https://doi.org/10.1287/mksc.2018.1095
- Sydler, R., Haefliger, S., & Pruksa, R. (2014). Measuring intellectual capital with financial figures: Can we predict firm profitability? European Management Journal. https://doi.org/10.1016/j.emj.2013.01.008
- Teeter, P., & Sandberg, J. (2017). Cracking the enigma of asset bubbles with narratives. Strategic Organization, 15(1), 91–99. https://doi.org/10.1177/1476127016629880
- The Economist. (2017). Google leads in the race to dominate artificial intelligence - Battle of the brains. Retrieved December 12, 2018, from https://www.economist.com/business/2017/12/07/google-leads-in-the-race-to-dominate-artificial-intelligence
- The White House (2018). Summary of the 2018 White House Summit on Artificial Intelligence for Amircan Industry. Washington, D.C.
- U.S. Department of Labor. (1999). Issues in Labor Statistics: Computer Ownership Up Sharply in the 1990s. Retrieved from http://www.bls.gov/cpi
- Venture Scanner. (2018). Artificial Intelligence Startup Highlights – Q3 2018. Retrieved December 12, 2018, from https://www.venturescanner.com/blog/2018/artificial-intelligence-startup-highlights-q3-2018
- Walch, K. (2018). Is Venture Capital investment in AI Realistic or Out of Control? Retrieved December 12, 2018, from https://medium.com/cognilytica/is-venture-capital-investment-in-ai-realistic-or-out-of-control-cb46b428590
- Zhang, R. (2015). Ambiguity Aversion and the Stock Market Participation: Empirical Evidence. University of Exeter.
Write a comment